Towards interpretable speech biomarkers: exploring MFCCs
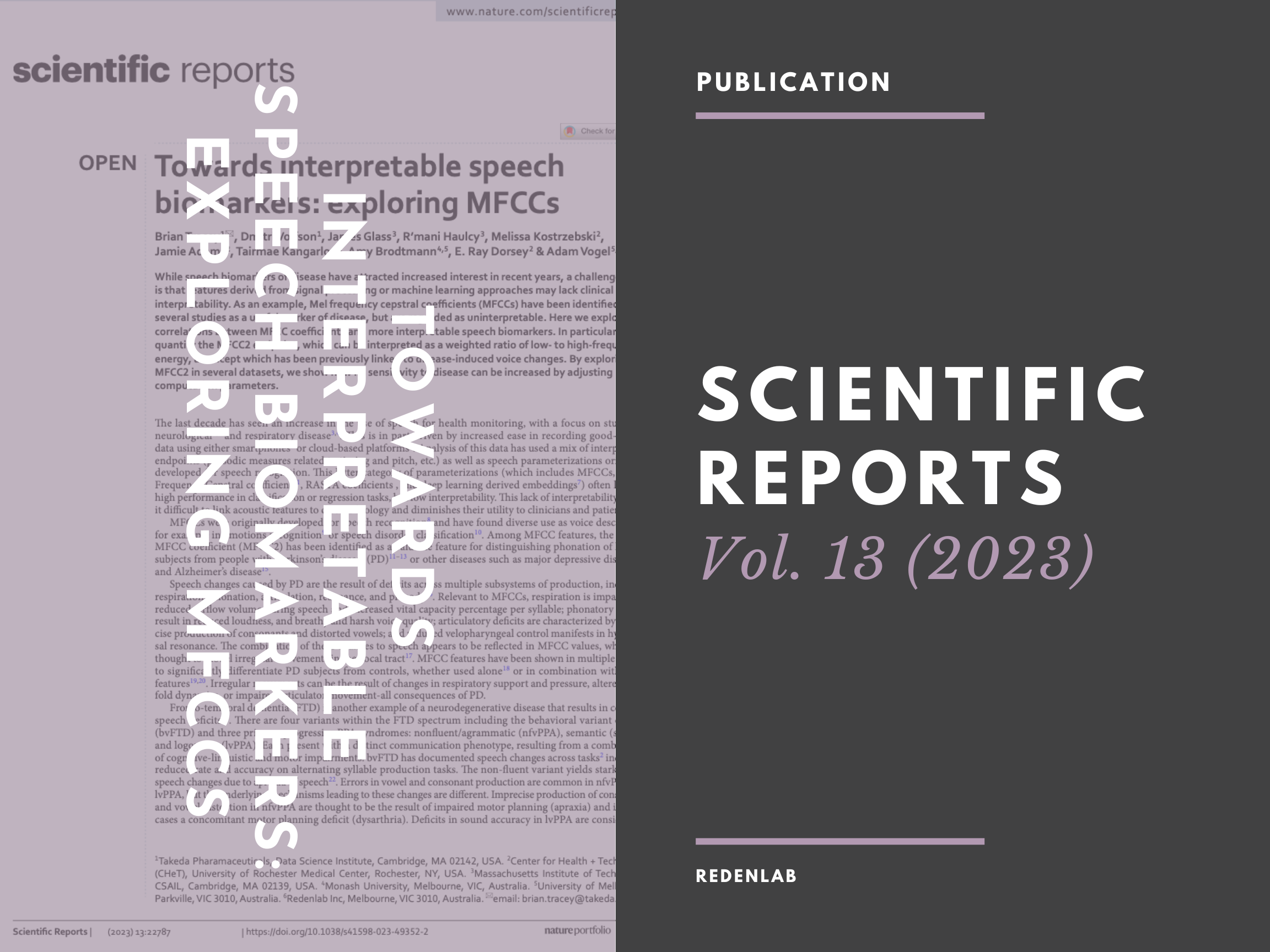
While speech biomarkers of disease have attracted increased interest in recent years, a challenge is that features derived from signal processing or machine learning approaches may lack clinical interpretability. This study examined a commonly used feature, Mel Frequency Cepstral Coefficient 2 (MFCC), in two progressive brain diseases, Frontotemporal dementia and Parkinson’s disease. Data show sensitivity to disease of this feature can be increased by adjusting computation parameters.
This study was conducted in collaboration with Takeda, University of Melbourne, MIT, Monash and University of Rochester Medical Center.
To find out more about the study, click here.